- July 9, 2021
- Posted by: BJ Hudson
- Categories: Data, Economics, Innovation, Security, Technology
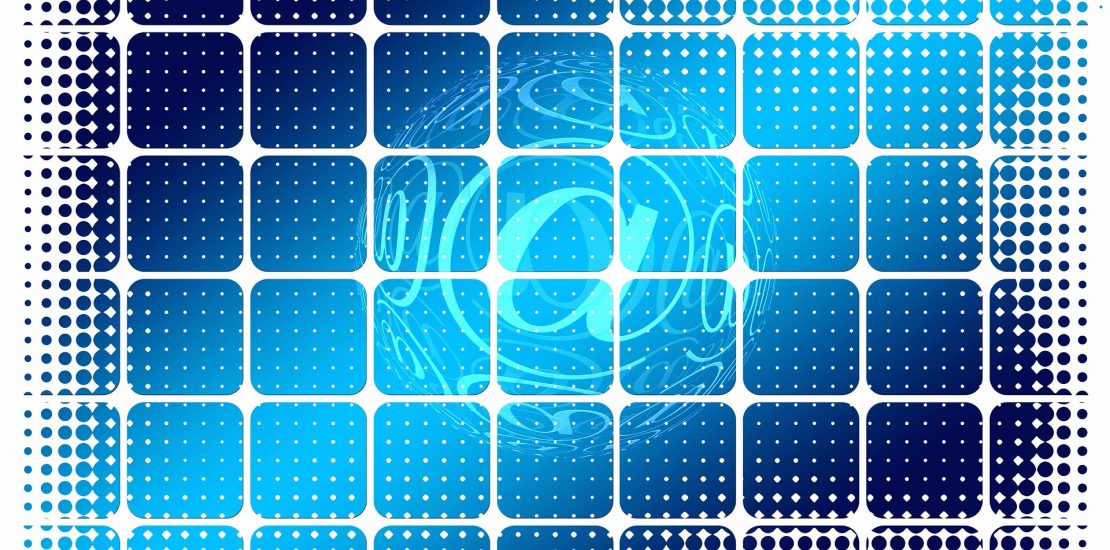
Written by Dave Nyczepir
JUL 2, 2021 | FEDSCOOP
The Government Accountability Office has released its much-anticipated artificial intelligence accountability framework in an effort to oversee how agencies are implementing the emerging technology.
GAO‘s framework describes key practices across four parts of the development life cycle — governance, data, performance and monitoring — to help agencies, industry, academia and nonprofits responsibly deploy AI.
Agencies’ inspectors general (IGs), legal counsels, auditors and other compliance professionals needed a framework to conduct their own credible assessments of AI notwithstanding congressional audit requests.
“The only way for you to verify that your AI, in fact, is not biased is through independent verification, and that piece of the conversation has been largely missing,” Taka Ariga, chief data scientist at GAO, told FedScoop. “So GAO, given our oversight role, decided to take a proactive step in filling that gap and not necessarily wait for some technology maturity plateau before we addressed it.”
GAO would always be playing catch-up, given the speed AI is advancing, otherwise, Ariga added.
AI systems are made up of components like machine-learning models that must operate according to the same mission values. For instance, self-driving cars with their cameras and computer vision are systems of systems all working to ensure passenger safety, and it falls not only to auditors but ethicists and civil liberties groups to discuss both their performance and societal impacts.
“We want to make sure that oversight is not being treated as a compliance function,” Ariga said. “There are complicated risks around privacy, complicated risks around technology, around procurement and around disparate impacts.”
GAO’s framework, released Wednesday, is a “forward-looking” way to address those risks absent a standard risk-management framework specific to AI, he added. The agency wants to ensure risk management, oversight and implementation co-evolve as the technology advances to what the Defense Advanced Research Projects Agency calls Wave 3: contextual adaptation, where AI models explain their decisions to drive further decisions.
Another goal of the framework is to include a human-centered element in AI deployment.
With agencies already procuring AI solutions, GAO’s framework makes requirements, documentation and evaluation inherently governmental functions. That’s why every practice outlined includes a set of questions for oversight bodies, auditors and third-party assessors to ask, in addition to procedures for the latter two groups.
The rights to audit AI, inspect models and access data are critical to their efforts.
“It will be detrimental long term if vendors are able to shield the intellectual property aspects of the conversation,” Ariga said.
Attempts to audit AI have already occurred, most notably the Department of Defense‘s effort when the Joint AI Center was created in 2018. But DOD ran into issues because there was no standard definition of AI, and it lacked AI inventories to assess. Fast forward to the present day, and many companies now offer AI and algorithmic assessments.
GAO is already using its new framework to investigate various AI use cases, and other agencies’ IGs have expressed interest in using it, too.
“The timing is great because we actually have a number of ongoing engagements in national security, in homeland security, in the justice domain that involve AI,” Ariga said.
The framework will evolve over time, possibly into an AI scorecard for agencies — an idea proposed by former Rep. Will Hurd, R-Texas, in September.
Google and the JAIC are considering AI model or data cards, while nonprofits have proposed something more akin to a nutrition label, but GAO’s framework doesn’t prescribe a particular accountability method— rather it evaluates the rationale behind whatever mechanism is chosen.
Future iterations of the framework will also ask what transparency and explainability mean for different AI use cases. From facial recognition to self-driving cars to application-screening algorithms to drug development, each carries with it varying degrees of privacy and technology risk.
People won’t need a justification for every turn a self-driving car makes, but they’ll eventually want to know why, to the nth degree, and algorithm is flagging an MRI as anomalous in a cancer diagnosis.
“We knew having to do individual use case nuances would’ve taken us decades before we could ever issue something like this,” Ariga said. “So we decided to focus on common elements of all AI development.”
At the same time departments like Transportation and Veterans Affairs have started collaborating to develop their AI strategies, even though the former’s focus is safety and the latter’s customer service — given their shared workforce, infrastructure, development and procurement issues.
In developing the framework, Ariga said he was “surprised” to find not everyone in government is on board with the notion of accountable AI.
Undergraduate data scientists don’t always receive ethics training and are instead taught to prioritize accuracy, performance and confidence. They carry that perspective with them into government jobs developing AI code, only to have people tell them to eliminate bias for the first time, Ariga said.
At the same time a competing camp argues data scientists shouldn’t shape the world that should be but reflect the one they live in, and AI bias and disparate impacts are someone else’s problem.
Ariga’s team kept that disagreement in mind, while engaging with government and industry AI experts and oversight officials, to avoid placing an undue onus on any one group while developing GAO’s framework.
Government will eventually need to provide additional AI ethics training to data scientists as part of workforce and implementation risk management, training that academic institutions will likely adopt — much the same way medical ethicists came about, Ariga said.
“Maybe not tomorrow but certainly in the near future because, at least in the public sector domain, our responsibility to get it right is so high,” he said. “A lot of these AI implementations actually do have life or death consequences.”